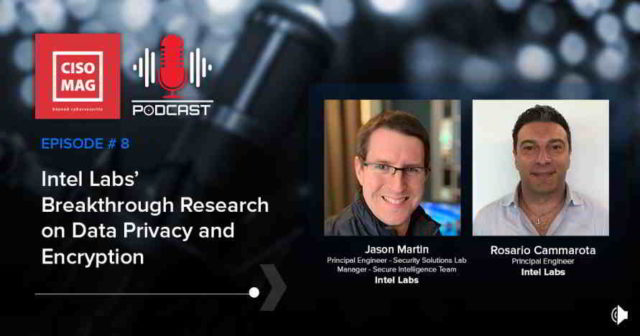
Data privacy is now a big concern, not just for individuals, but also for organizations and governments. There’s data at rest, in transit, and while it is being processed. Now we know that it can be encrypted at rest or in transit. But it must be decrypted for processing. And that window is an opportunity for the bad guys to come in and steal data or change it. So, it becomes mutable.
Researchers at Intel Labs have found a way to process encrypted data sets without the need to first decrypt them. However, there are a few challenges to overcome before the technology becomes mainstream. Homomorphic Encryption is a new cryptosystem that allows applications to perform computation directly on encrypted data, without exposing the data itself. The technology is emerging as a leading method to protect the privacy of data when delegating computation.
Federated Learning can solve the challenges of sharing large data sets between entities. It uses machine learning tools to offer valuable insights from the data.
In this episode, we have Rosario Cammarota (“Ro”) – Principal Engineer at Intel Labs, and Jason Martin – Principal Engineer in the Security Solutions Lab and manager of the Secure Intelligence Team at Intel Labs. They explain how Federated Learning and Homomorphic Encryption are solving data challenges in health care research – and the possible applications in other industries.
RSS: https://feeds.soundcloud.com/users/soundcloud:users:899202688/sounds.rss
Spotify: https://open.spotify.com/show/7pBhvwEVAaL4uUJnzD5rWO
Jason Martin is a Principal Engineer in the Security Solutions Lab and manager of the Secure Intelligence Team at Intel Labs. He leads a team of diverse researchers to investigate machine learning security and privacy in a way that incorporates the latest research findings and Intel products. Jason’s interests include machine learning, authentication and identity, trusted execution technology, wearable computing, mobile security, and privacy. Prior to Intel Labs, he spent several years as a security researcher performing security evaluations and penetration tests on Intel’s products.
Rosario Cammarota (“Ro”) is a Principal Engineer at Intel Labs, where he leads the effort on privacy-enhancing cryptographic technologies, their application, and standardization. He received his Ph.D. degree in Computer Science from the University of California, Irvine.
Ro’s research is at the intersection between cryptography and computing, focusing on fully homomorphic encryption, secure multi-party computation, and their application to artificial intelligence and statistics. Furthermore, his research interests include hardware and system security.
Ro is one of the technical advisory board members at the Semiconductor Research Corporation (SRC), where he contributes to developing research programs in hardware security and artificial intelligence hardware. In this role, he acts as a technology transfer facilitator. He is one of the US-experts at the International Organization for Standardization (ISO), where he contributes to developing standards on trustworthiness in artificial intelligence and privacy-enhancing technologies.
Ro is a Senior Member of IEEE. He is a prolific author and inventor. His research appears in journals and conferences such as ACM TECS, DAC, IEEE HOST, among others. He is one of the recipients of the SRC Outstanding Industry Liaison Awards in 2017, 2018, and 2019.
Listen to our previous podcast episodes here.
About the Host
Brian Pereira is the Editor-in-Chief of CISO MAG. He has been writing on business technology concepts for the past 26 years.