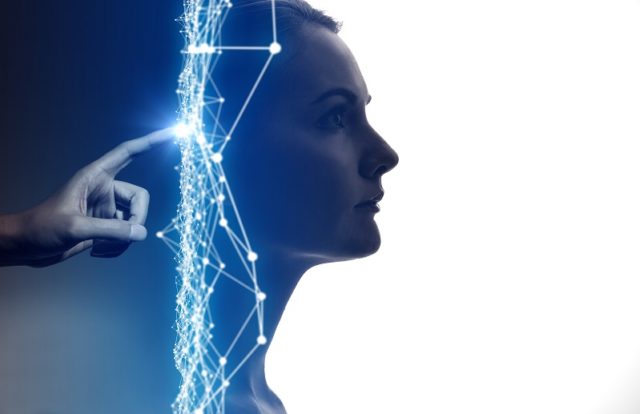
In 2004, a few unmanned vehicles showed up at the starting gate of the lengthy course across the Mojave Desert — this was the inaugural DARPA Grand Challenge. It signified the beginning of the technological race to develop a practical self-driving car, which sparked a global movement that continues even today.
The networking community too embarked on a similar journey to provide production-ready, economically feasible, Self-Driving Networks. Self-Driving Networks are autonomous networks that use Artificial Intelligence (AI) and Machine Learning (ML) to program independently and carry out prescribed intentions while eliminating complex programming and management tasks required today to run the networks. In view of this, the proliferation of data breaches and cyberattacks in today’s networking environment has also increased, leading to extensive repercussions across businesses. As such, ML-based security solutions have become a major cybersecurity investment for organizations today.
By Rohit Sawhney Systems Engineering Manager at Juniper Networks India
Leveraging AI to enhance your network security
Many experts believe that AI and ML will dominate cybersecurity in the future. Last year, at the Gartner IT Symposium/Xpo, analysts discussed how these two technologies will augment human decision-making, emotions, and relationships.
Rapid technological advances are enabling AI to disrupt the networking industry with new insights and automation. AI in the networking domain will be able to reduce IT costs and offer the best possible user experience. Not only will AI be able to reduce IT costs, but it will also bring in more productivity and efficiency in networking. Together, machine learning and AI could be key enablers, helping to reduce human effort and make cybersecurity faster, more consistent and accurate.
In fact, many Enterprises are already making greater investments to integrate solutions with machine learning algorithms into their existing security infrastructure. While traditional antivirus programs are still widely used to detect and neutralize threats, they do not have the capability to detect and mitigate sophisticated threats. ML-based security solutions like the Juniper ATP can help monitor potential threats in the network through threat intelligence features – allowing IT security teams to detect any suspicious activity before the attack occurs.
AI comes to the rescue as it reduces the number of monotonous tasks that take up an engineer’s time, while ensuring they are always completed accurately, regardless of frequency and quantity. This allows engineers to focus on other business strategic tasks while maintaining network health and safety.
Building an AI system for your network
In a recent survey conducted by KPMG for its report, Living in an AI World 2020, analysts found that 92% of respondents agree that leveraging spectrum of AI technologies will make their companies run more efficiently. However, in the networking domain, IT simply can’t meet the needs of today’s stringent network requirements, without a robust AI strategy. The following are some technology elements that an AI strategy should include:
- Data – Needless to say, without adequate and relevant data, ML algorithms are as good as the data one ingests in them. The more diverse the data collected, the smarter the AI solution becomes. The collection of real-time data with accuracy and speed is just as important. Edge devices like routers, mobiles, and IoT enabled solutions not only need to collect the data but get it processed quickly in a nearby edge computer or on the cloud, using AI algorithms, to make the network more adaptive.
- Domain-specific expertise – Unless you are a domain expert, it is simply impossible to replicate an AI system to diagnose wireless problems. Placing the metadata at the center of these problems and breaking it into small fractions will enable the AI system to understand the complexity and get trained.
- Data science deep dive – Machine Learning and Big Data techniques empower the data by extracting insights from the multiple chunks of metadata, divided into several domains.
- Virtual network assistants – When Netflix or Prime Video recommends movies basis the ones you’ve been watching, they’re using an ML technique known as collaborative filtering. Apart from recommendations, collaborative filtering can also be applied to sieve through large data sets and identify and correlate those that form an AI solution to a problem.
Benefits of AI/ML in the networking domain
ML a subset of AI, is a prerequisite for any successful deployment of AI technologies. ML uses algorithms to parse data, learn from it, and determines or predicts without requiring explicit instructions. With that said, AI/ML can be leveraged for the following tasks in the networking domain:
- Cybersecurity practices: Machine Learning is critical in building a secure networking infrastructure and is considered a top priority for most organizations today. ML enables security automation and helps in data classification, processing, filtering, and significantly managing and reducing the workload of the IT security team. Automating this task increases workload efficiency and reduces the risk of missing an important threat alert.
- Predict user experience to dynamically adjust bandwidth demand – When traffic spikes occur in today’s networks, it is difficult to distinguish between DDoS attacks from widespread downloading, of say, Arijit Singh’s latest album. By leveraging ML algorithms that interpret copious amounts of traffic behavior data, the Self-Driving Network will be able to predict performance issues before users are affected. In such an example, connections with algorithms that scrape Twitter feeds will confirm the hypothesis: Have hacking groups been threatening action against an enterprise? Or have fans been demanding for Arijit Singh’s album in the weeks leading up to the spike? The Self-Driving Network will analyze and adapt accordingly, either shutting down ports to isolate the DDoS attack or adding bandwidth to accommodate the surge in album downloads.
- Self-correct for maximum uptime – AI, through its intelligent algorithms, complemented by ML capabilities, enable systems to have a self-correction process in places to ensure maximum uptime. The powerful AI-driven networks can even capture data prior to a network event or outage, which accelerates troubleshooting.
- Instantly find root causes – AI can leverage multiple data-mining techniques to explore terabytes of data in a matter of minutes. This allows IT departments to instantly identify what network feature – be it the OS, device type, access point or switch – is most related to a network problem. This in turn allows IT departments to accelerate this problem resolution.
About the Author
Rohit Sawhney is a Systems Engineering Manager at Juniper Networks India. He leads the team of Technical Consultants supporting Juniper’s North/East India & SAARC business. Prior to joining Juniper Networks, he has worked with IBM India and has industry experience of over 20 years. Rohit is a certified by Juniper Networks, Cisco and VMWare. He holds a master’s degree in Computer Application from Sikkim Manipal University of Health, Medical and Technological Sciences and a Bachelor’s of Science in Electronics from Delhi University.
Disclaimer
CISO MAG did not evaluate/test the products mentioned in this article, nor does it endorse any of the claims made by the writer. The facts, opinions, and language in the article do not reflect the views of CISO MAG and CISO MAG does not assume any responsibility or liability for the same. CISO MAG does not guarantee the satisfactory performance of the products mentioned in this article.