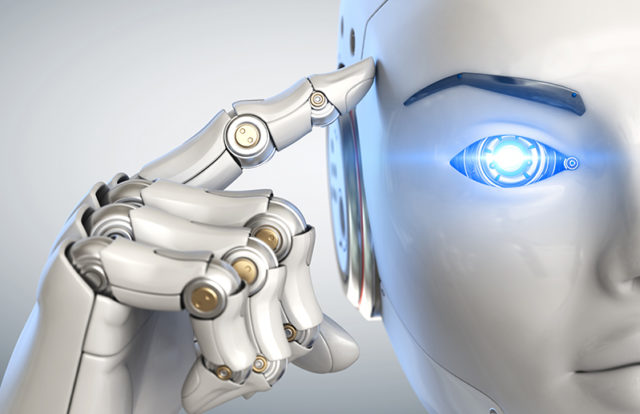
Many believe that cybersecurity is an exciting field to work in, and indeed it is. Yet being responsible for an organization’s IT Security is no easy feat. Attackers always seem to be a few steps ahead of defenders. It often feels like a game of one against many – from petty criminals to nation-states. It would be highly advantageous if our cybersecurity tools could automatically adapt to these threats. The good news is that security vendors are increasingly promising exactly this; machine learning (ML) and artificial intelligence (AI) will supposedly solve all our problems through automatic adaptation.
By Dr. Serge Droz, Chair, Forum of Incident Response and Security Teams (FIRST), and Senior Advisor at ICT4Peace
What is AI?
The term goes back to a workshop at Dartmouth College held in 1956. However, today, roughly speaking, it leverages two mathematical disciplines – statistics methods and neural networks.
A good example of the former is Bayesian email spam filters – the statistical distribution of words in each message is calculated and compared to a number obtained from a corpus of legitimate and spam messages. The filters typically require access to large amounts of data before making meaningful predictions, which can become challenging. This is the reason that large mail providers, with access to millions of messages, have a much higher success of correctly classifying messages when users are also helping to tag spam messages.
Neural networks on the other hand are loosely inspired by the human brain; in a training phase, connections between strands of the network are adjusted to maximize a certain value function. No one understands what exactly happens in such a network, but they are very successful at recognizing patterns.
Access to curated training data is crucial for the proper functioning of these methods. This sounds easier than it is. Not only is a lot of data needed, but it must also be of good quality. Any error, or bias, in the training data will re-emerge in the classification, producing false positives and false negatives. A good example of this is face recognition. Most commercially available products have been trained on collecting images based on where the products are engineered. This has resulted in white males being accurately identified 99.5 % but falls way below 70% for women of color. Obviously, this is a problem when such algorithms are used in consequential decision making such as unlocking a phone or granting access to a secure facility, Examples like these are ample. But image recognition has been stunningly successful in some areas, e.g., medical diagnostics. One of the reasons is that most medical imagery is extremely well classified.
So, what is the reality for cybersecurity?
Traditionally security tools have been based on signatures – clear markers of malicious activity. Let us focus on one example for the moment. A classic example is virus scanners which look for unique characteristics in pieces of code. However, this method is becoming increasingly more difficult with the ever-increasing amount of malware – AV signatures are often updated several times per day. This is similar to the biological world – the flu virus is very adaptable, so the human immune system constantly needs to adapt to new versions of the flu.
So, could AI recognize generic patterns of malware? Indeed, most AV products today seem to contain AI and ML. Unfortunately, many of these algorithms are too naive and perform poorly under real-life conditions due to a bad understanding of the data on one hand and encryption on the other.
Classifying cat pictures by using pictures with cats, rather than pictures of cats will likely fail. It cannot be reiterated enough: training data must be of good quality. In recent years, however, people have begun to train classifiers on components of disassembled malware. And indeed, this seems to be a much more promising approach. It however requires a more detailed look at samples and an understanding of program code. Naively applying AI to blobs of data doesn’t work. This is tied to the second stumbling stone – encryption. Good encryption removes the statistical properties of the original data. Statistical classification will thus fail for exactly this reason. Malware authors today routinely encrypt and pack in the jargon to make the analysis of their malware more difficult.
Other applications
Machine learning (ML) or AI is generally useful when searching for complex patterns in large amounts of data. Typically, security specialists want to find hints of breaches and at the same time reduce the number of false positives. Breaches are, despite all, very rare compared to the many legitimate events making them difficult to spot by statistical methods. People have applied ML techniques to network anomalies, but with little success so far. Another area that seems to be popular is UEBA, User and Entity-based Behavior. This looks at the fact that attackers exhibit different behaviors from regular users. Unfortunately, regular users can behave in an extremely diverse fashion, so an action can only be labeled legitimate by evaluating the context of the action. This information gathering can be automated and runs under the term security orchestration.
A way forward
Today AI has very limited applications in cybersecurity. AI also has dangers, in particular bias.
AI works reasonably well with large amounts of data, but only a few organizations have an adequate volume for AI to be useful. However, the AI field is evolving rapidly, and it is certainly worth keeping an eye on new developments, some of which cannot be anticipated. Research is often not linear – it may well be that new paradigms will help solve some of the intractable problems. But more importantly, it’s too early to say goodbye to traditional signature-based detection methods. The bulk of cyber threats are still recognized using signatures, and new standards such as Yara and Sigma rules have moved the field forward. And interesting projects are trying to combine signature-based detection with AI.
It’s important to understand the underlying methodology when investigating AI solutions for your organization. Vendors need to be more transparent about what their AI solutions do behind the scenes. At the same time organizations need to invest more resources into understanding their data to profit from it, security or otherwise. Just collecting data and hoping a magical algorithm finds the golden needle may work in movies, but rarely works in reality.
About the Author
Dr. Serge Droz is a senior IT-Security expert and seasoned incident responder working at Proton Technologies. He studied physics at ETH Zurich and the University of Alberta, Canada, and holds a Ph.D. in theoretical astrophysics. He has worked in private industry and academia in Switzerland and Canada, among others as a Chief Security Officer of Paul Scherrer Institute, as well as in different security roles at the national CERT in Switzerland for more than 15 years. Serge is the chair of the board of directors of FIRST (Forum for Incident Response and Security Teams), the premier organization of recognized global leaders in incident response, and a Senior Advisor to the Swiss-based ICT4Peace foundation. He also served for two years in the ENISA (European Union Agency for Network and Information Security) permanent stakeholder group. Serge is an active speaker and a regular trainer for CSIRT (Computer Security Incident Response Team) courses around the world.
Disclaimer
Views expressed in this article are personal. The facts, opinions, and language in the article do not reflect the views of CISO MAG and CISO MAG does not assume any responsibility or liability for the same.