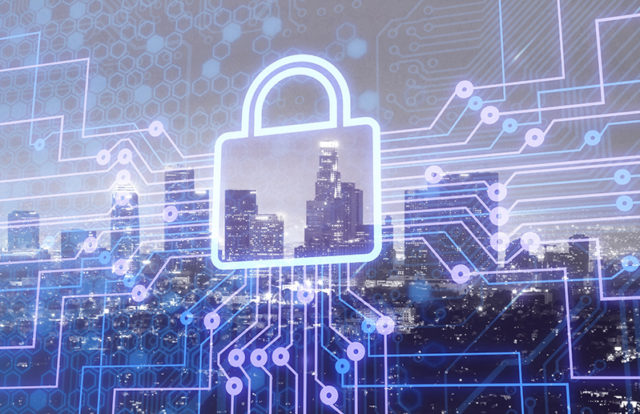
Contributed By Oren Yunger, Investor at GGV Capital, and Omer Singer, Senior Director of Security at Snowflake
For every hour that security teams spend triaging alerts, how many minutes do they spend applying their cybersecurity expertise? In our experience, it’s only a small fraction of the time. Why such a ratio? The complexity of their environment requires analysts to rely on intimate familiarity with the organization to determine whether an alert represents an actual breach. In this article, we’ll share how data-driven security teams are breaking down data silos, improving alerts telemetry and enjoying a 90% reduction of false positive alerts.
Most security professionals are probably familiar with Target’s classic example of alert triage failure. In 2013, Target’s Security Operations Center disregarded a generic alert that could have provided early warning of its massive data breach. Some observers might call out the SOC’s actions as negligent. But that would not be taking into account the challenge of dealing with thousands or millions of daily alerts in a complex and dynamic environment like that of Target.
While this challenge is well recognized, the prevailing approaches each suffer from major drawbacks.
Approach | Description | Drawbacks |
Hire more people | As data volumes increase, throw more bodies at the problem. Companies hire more analysts and gatekeepers to sift through false positives. | Many consider the skills shortage as the top challenge in cybersecurity. This approach raises questions about working harder vs. working smarter. |
Limit data scope | Drowning in alerts, security organizations avoid adding additional data sets. Security teams typically have a long wishlist of data sources that are blind spots “for now”. | Missed opportunities for effective threat detection and response. Might miss the one “slip up” that would have given the attackers away. |
Artificial Intelligence | Machine learning to the rescue, analyst robots will do the basic triage and correlation automatically. | Automated security analytics are not yet producing high fidelity, actionable results. Solutions tend to be limited in scope and user experience, with high levels of false positives and negatives. |
Security engineers and vendors should consider a fourth approach – adding more data into the mix. While too much data seemed to be a part of the problem, it turns out that more data can reduce noise for security teams that join together diverse data sets.
Consider a situation where an AWS user terminates ten Linux servers. A typical threat detection solution may alert on this anomaly as a possible indication of compromise, so that an analyst or automated playbook may disable the user and prevent further destruction. Triage is required before a decision can be made because there are a few possible explanations with very different outcomes.
One possible explanation is that a DevOps engineer was cleaning up old test servers that haven’t been used in a year. Alternatively, the same activity might be a compromised service account being hijacked to bring down core business servers during peak hours.
The correct triage depends on the context – there is a big difference between a DevOps engineer, who is a trusted privileged user, operating from the office with a company managed device, authenticated through Okta, as opposed to a recently terminated IT admin who’s logging in from Starbucks.
Understanding these differences makes up most of the time that people spend on alert triage. Automatically differentiating between those two scenarios requires additional data beyond the activity logs. The additional data must provide context to the decision making, changing the logic from a single dimension (“what happened”) to multiple dimensions, which include user context (“who is this user”) and asset context (“what is this system”).
As the field of security analytics matures, contextual information is increasingly applied to the alert logic. Multi-dimensional detection logic can avoid raising alerts that would be disregarded by analysts. Even better, breaches that would otherwise be missed can be surfaced to security teams in time.
For example, resources in AWS may be tagged by role: production, sandbox, etc. While AWS CloudTrail activity logs do not include these tags, detection logic that can access stored instance configuration data can avoid alerts for sandbox resources and elevate severity on production ones. Another application for context can be HR data – even routine activity by someone that’s been recently fired or given notice of termination should set off alarm bells.
The catch? The correlations described above require analyzing data that is not found in most organizations’ SIEM solutions or security data lakes. As such, security teams looking to cut noise and improve detection capabilities through better security analytics need to start by getting the data into a single, queryable location. For many of these data sources, that means reaching across the organization to teams that have never before thought of their data as “security data”.
Examples of data sources that can enrich context and improve alert fidelity include:
- Human Resources (HR) records with data on employee termination
- User directory records with data on employee team affiliation
- Endpoint activity logs describing what was running on the laptop of an admin while their user was making changes to the cloud environment
- Email activity logs indicating possible phishing prior to unusual user activity
- Vulnerability management findings for at-risk servers
- AWS tag data linking resources to environments and data classifications
Unfortunately, not all of this information is readily interconnected and accessible for security teams today. Let’s dive into what analytics stack is available for organizations that are looking to tap into the potential of contextual data for improving their security analytics. Some threat detection solutions are optimized for search rather than joining myriad datasets. Traditional SIEM solutions, such as HP’s ArcSight, support correlation along a single dimension but suffer from performance limitations on joins and actually warn users against going beyond very limited data joining. These limitations prevent the needed contextual enrichment. For that purpose, modern data warehouses are increasingly becoming the platform for multi-dimensional threat detection and response, the kind of “connecting the dots” that frees up analysts and catches intrusions that would have otherwise been missed.
Not all organizations will be able to go beyond aggregating data to their data warehouse. For more advanced threat detection and mitigation capabilities, emerging vendors combine existing datasets to provide actionable insights. A new breed of security startups is focusing on effective analytics instead of adding more sensors. Customers should ask security vendors to demonstrate that they are able to combine multiple datasets to see the full picture and achieve high-fidelity results.
Even with access to a modern data warehouse, many security teams may not be able to create the analytics and correlation needed to take advantage of the technology. There is a great amount of knowledge siloed across different organizations. This is a great opportunity for open source collaboration to lower the barriers and improve security teams telemetry. In the area of data connectors, open source can be used to standardize how event logs, asset inventory and configuration data is collected from the relatively short list of commonly used cloud-based sources. For connecting the dots among the same common sources, open source detection logic can be written, shared, and maintained by the community. The fact that JSON and SQL are standard across data sources and analytics systems means that rules can be vendor agnostic more than ever before.
To conclude, we believe that with a modern approach to data, security teams can move from a “more data equals more noise” paradigm to one where “more data equals more context and less noise”. Join this shift by initiating conversations within the organization to collect more internally available data as well as data from external third-parties. As customers, ask vendors to collect more contextual data and to use it to reduce false positives. Let’s encourage collaboration and knowledge sharing with like-minded security teams, and make our industry a less noisy place to do good security.
The opinions expressed in this article are the personal opinions of the author. The facts and opinions appearing in the article do not reflect the views of CISO MAG and CISO MAG does not assume any responsibility or liability for the same.