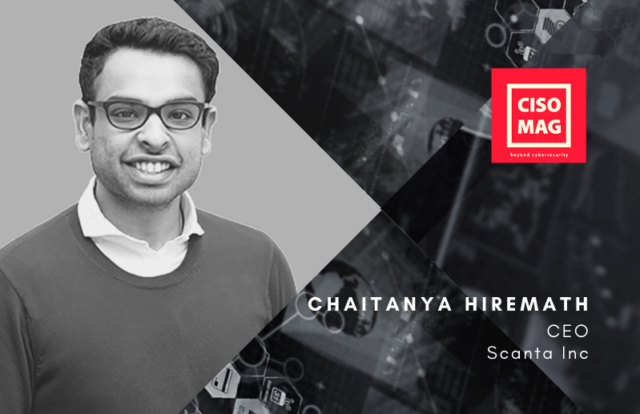
Chaitanya Hiremath is the CEO of San Francisco-based AI firm, Scanta Inc. He is the first Indian-origin entrepreneur to win the Shark Tank Showcase and the prestigious Draper University ‘Summer Pitch’ in 2018 in San Francisco. In 2019, he was listed in the ‘Top 25 People in Tech’ list by Entrepreneur Magazine, after also being chosen for the Forbes ‘30 Under 30’ list, in the Startup category. He was named as the “Youth Icon” for 2019 by Fame India. He is a dynamic, results-oriented leader with a strong track record in cutting-edge technologies at fast-paced organizations. His expertise in artificial intelligence, machine learning, and cybersecurity has helped him build a worldwide team of innovators at Scanta. His latest challenge has been to develop market-leading security technology to protect (Virtual Assistant) VA Chatbots.
In an exclusive interview with Augustin Kurian from CISO MAG, Chaitanya talks about his journey, the future of chatbots, and security.
What was the problem with ML that you have set out to fix? How often are VA (virtual assisted) chatbots being targeted in cyberattacks? What kinds of damages can an unprotected ML-powered VA system cause to an organization?
Interestingly enough I think the basic thesis here is that you are working on the larger vision of protecting machine learning systems, and when you are looking at how you use machine learning systems as a use case, the first risk which has a lot of adoption was virtual assistant chatbots. That’s because machine learning is a vast ocean of use cases. So, when we hoard it in VA chatbots, there are different vulnerabilities that arise from it.
I was working on a larger thesis of what makes a machine learning system vulnerable. It’s just a simple process if you look at it, I can give you an example of Tesla self-driving cars. Tesla self-driving cars are able to classify a “STOP” sign or a “GREEN” light and make decisions based on the interpretations. What happened last year was shocking, which also compelled us to begin looking into this space. It led to people losing their lives. In this scenario, one of Tesla’s self-driving cars was operating in autopilot mode and did not interpret the “STOP” sign, which led to a car crash and killed the driver. Everybody looked at this problem saying that this is a one in a million case, something like this happens if a machine learning system is not able to detect the signs.
But actually, it was much deeper than that. Somebody had attacked the system externally, not internally. We would normally interpret the Stop sign with red-colored letters “S.T.O.P,” right? However, for a machine learning system, Stop, signs are based on patterns. Similarly, in Tesla’s case, somebody put a tape on top of the Stop sign. Hence, the system classified the Stop sign as a green light and the car went on driving autonomously and failed to recognize the sign. If you look at this, this has mass repercussions. If somebody can misclassify a decision by just adding tape, it is like scratching the tip of the iceberg of what’s really with machine learning systems. This is a problem in ML systems and particularly with VA Chatbots.
Well, now that we have discussed threats vectors and this is a new niche, we can assume VA has been occupying a special niche in the global IoT ecosystem. They have been playing a major part in home automation as well. Do you think a cyberattack on VA can have a domino effect at multiple vectors across the entire line-up?
Yes, and it is simple, specifically with the IoT devices. They don’t have any security embedded in them. They lack layers of protection and they don’t have any authentication or verification. These systems are particularly vulnerable because they all work in a network. If I’m attacking an IoT system somewhere around San Francisco, I can get on a system anywhere. It’s based on the network effect. It is not only confined to somebody getting into the system but once somebody is in the system, there are thousands of ways in which it can impact. Just to give you an example about interacting and sharing confidential information, which people always do these days. If you have an app on top of Alexa or anything else, you can possibly extract that information out easily if it is left unprotected.
These are sophisticated mechanisms but they are more than possible.
There’s a massive demand for VA (virtual assistants) around the world, but one of the things that have caused many vulnerabilities is that organizations usually find it difficult to balance between the coolness of the VA/VR features and the security, often making security an afterthought. Do you agree that even when the world is so much aware of cybersecurity, a lot of makers of VA chatbots are considering security as an afterthought?
Interesting question! So, you are basically saying that people are focusing too much on the coolness factor rather than the security aspect. The issue is that you can make your chatbot or VA as cool as possible. It is subjective but at the same time even if it has all the trendy features it doesn’t matter because according to us nobody is focusing on security. At least the ones we have spoken to, and we have spoken to few Fortune 500 companies, but nobody has been protecting the chatbots at a level we are talking about and it’s very different to protect a chatbot through a firewall because these are not correlated things. Chatbots are vulnerable at the conversational level and not just at the HTTP level, and that is the difference that we are trying to drive here.
Irrespective of how you set up the security architecture or you think you have a completely safe system, the training data set, all in-house and not open source, it still doesn’t matter. It’s still possible for anybody to get into the system and try to extract information or at the backend manipulate it in such a way that it does activities that you are not aware of, or that are adversarial in nature.
Do you think attacks against VA chatbots is getting its due share of importance? Are CISOs considering attacks against chatbots as an emerging threat? How can CISOs be instrumental in averting this threat vector? What best practices should CISOs have in place?
I have spoken to at least 50 CISOs and CIOs, some from Fortune 500 companies. Apart from two or three CISOs/CIOs, none of them were aware of this threat vector at all. So, the first reaction to “the chatbot can be attacked” is “Oh My God! I didn’t really know that we have to protect this also now.” Well, you have to. If you are making sure that your data is protected and spending millions of dollars for smooth functioning, then it is your responsibility to make sure that nothing can be extracted from the chatbot as well. I mean it is as important, right? You cannot protect yourself at the data level or the public/private cloud or whatever you have set up; it expands to more than that.
If somebody can directly extract the information from the chatbot, then that’s an issue and I haven’t really seen many CIOs or CISOs being aware of this threat vector. So firstly, it’s about being aware even beyond chatbots. If you are using anything in ML, the data can be poisoned and one way to really look at this is to know that more than 80% of all chatbots are built on an open-source training data set or an open-source algorithm, and you need to make sure that if you are making anything on open source, then you don’t take that as faith value. There have been examples of back-door channels with malicious codes inside the algorithm, so make sure you are betting this to the best of your capabilities when you are taking anything from the open-source. And the last aspect, which is sort of my recommendation, is when you are setting up the architecture itself you can protect it in a certain way but again, on the chatbot side particularly, that is something that the critical aspect is to analyze to see what goes in and comes out of the chatbot.
One more question, about the knowledge of the vendor. How often do you think vendors realize the need for security updates of their devices’ firmware on a regular basis? Because that was something Amazon had to focus on after the discovery of the KRACK bug. How much of this entire role is on the vendor’s side?
Well, the fundamental aspect here is that you need to be aware that the problem exists and the biggest issue that we face particularly in machine learning is that you may come across a case like Delta Air Lines six months down the line. One of the aspects here is to ensure some sort of Q&A is constantly added in your chatbot. Looking at where are different edge cases falling short—and that’s something vendors need to be really proactively on when these kinds of solutions are pushed into the market—but again it’s sort of a black box machine learning where we are not actually been able to know what kind of result you are getting, where is the request coming from, where is the response going. It’s an automated process, which is a common industry practice that is something we see changing now. We are getting numerous requests when we explain this new threat vector to people, we get a response like, “Oh it is something we are also affected by, or how can we check this?” If you deep dive into what goes in and comes out of the chatbot, it will give you a lot of exposure to where you stand with your security protocols.
About the Author
Augustin Kurian is part of the editorial team at CISO MAG and writes interviews and features.